목록전체 글 (20)
Hello Potato World
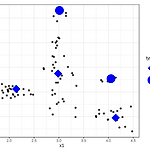
⋆ 。 ˚ ☁︎ ˚ 。 ⋆ 。 ˚ ☽ ˚ 。 ⋆ [XAI study_ Interpretable Machine Learning] 6.3 Prototypes and Criticisms Prototypes이란, 모든 데이터를 대표하는 data instance Criticisms란, Prototypes의 세트로 잘 표현되지 않는 data instance Prototypes와 Critisms은 Data를 설명하기 위해서도 사용되지만, Interpretable model을 만들거나 Black box 모델을 Interpretable하게 만들 때 사용될 수 있다. - Simulated Data Distribution Prototypes과 criticisms는 항상 데이터 내의 instances중 하나로 선택된다. (아..
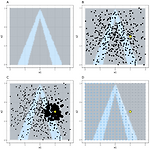
⋆ 。 ˚ ☁︎ ˚ 。 ⋆ 。 ˚ ☽ ˚ 。 ⋆ [XAI study_ Interpretable Machine Learning] 5.7 Local Surrogate (LIME) Local Surrogate Model이란? Black box 모델의 개별 예측을 설명하는데 사용하는 해석 가능한 모델 - Local Interpretable Model-agnostic Explanations(LIME): 지역 대리 모델 제안 Global Surrogate 모델처럼 전체 Dataset을 사용하여 근사하는 것이 아니라, Black box 모델이 주어져서 Data Point을 원하는 만큼 입력하여 개별 예측을 얻어낼 수 있을 때, 그 Prediction들을 이해하는 것 - for prediction 변형된 sample들..
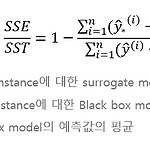
⋆ 。 ˚ ☁︎ ˚ 。 ⋆ 。 ˚ ☽ ˚ 。 ⋆ [XAI study_ Interpretable Machine Learning] 5.6 Global Surrogate Global Surrogate Model이란? Black box model의 예측에 근사하도록 학습된 interpretable model 아래와 같은 다양한 이름으로도 통한다 Surrogate(대체) Model = Approximation model = metamodel = response surgace model = emulator = ... Blackbox 모델, Surrogate 모델 둘 다 Machine Learning model Blackbox 모델의 예측에 최대한 근사 Surrogate 모델은 해석가능하여야 함 5.6.1 Theor..
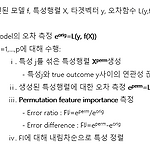
⋆ 。 ˚ ☁︎ ˚ 。 ⋆ 。 ˚ ☽ ˚ 。 ⋆ [XAI study_ Interpretable Machine Learning] 5.5 Permutation Feature Importance 특성 값을 섞은 후에 모델의 prediction error를 측정하여 구하는 방식 Important Feature feature의 값들을 섞었을 때 모델의 prediction error가 증가하는 경우 모델의 prediction이 특성에 의존적 Unimportant Feature feature의 값들을 섞었을 때 모델의 prediction error가 변하지 않는 경우 모델의 prediction이 특성에 무관 Algorithm The Permutation feature importance algorithm based ..
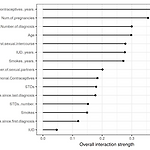
⋆ 。 ˚ ☁︎ ˚ 。 ⋆ 。 ˚ ☽ ˚ 。 ⋆ [XAI study_ Interpretable Machine Learning] 5.4 Feature Interaction prediction model 내에서 feature들이 상호작용하는 경우 각각의 feature effect의 합만으로는 예측을 표현할 수 없다. Linear Regression에서의 예시를 들어 확인해보자. 1. 두 개의 feature를 사용하는 linear regression 모델에서 feature interaction이 존재하지 않는 경우 X_1=1, X_2=1일 때의 data를 제외한 나머지 3개의 data를 사용하여 위와 같은 Linear Regression Function의 regression coefficient를 구해보면(e..